AI/ML

5 min read
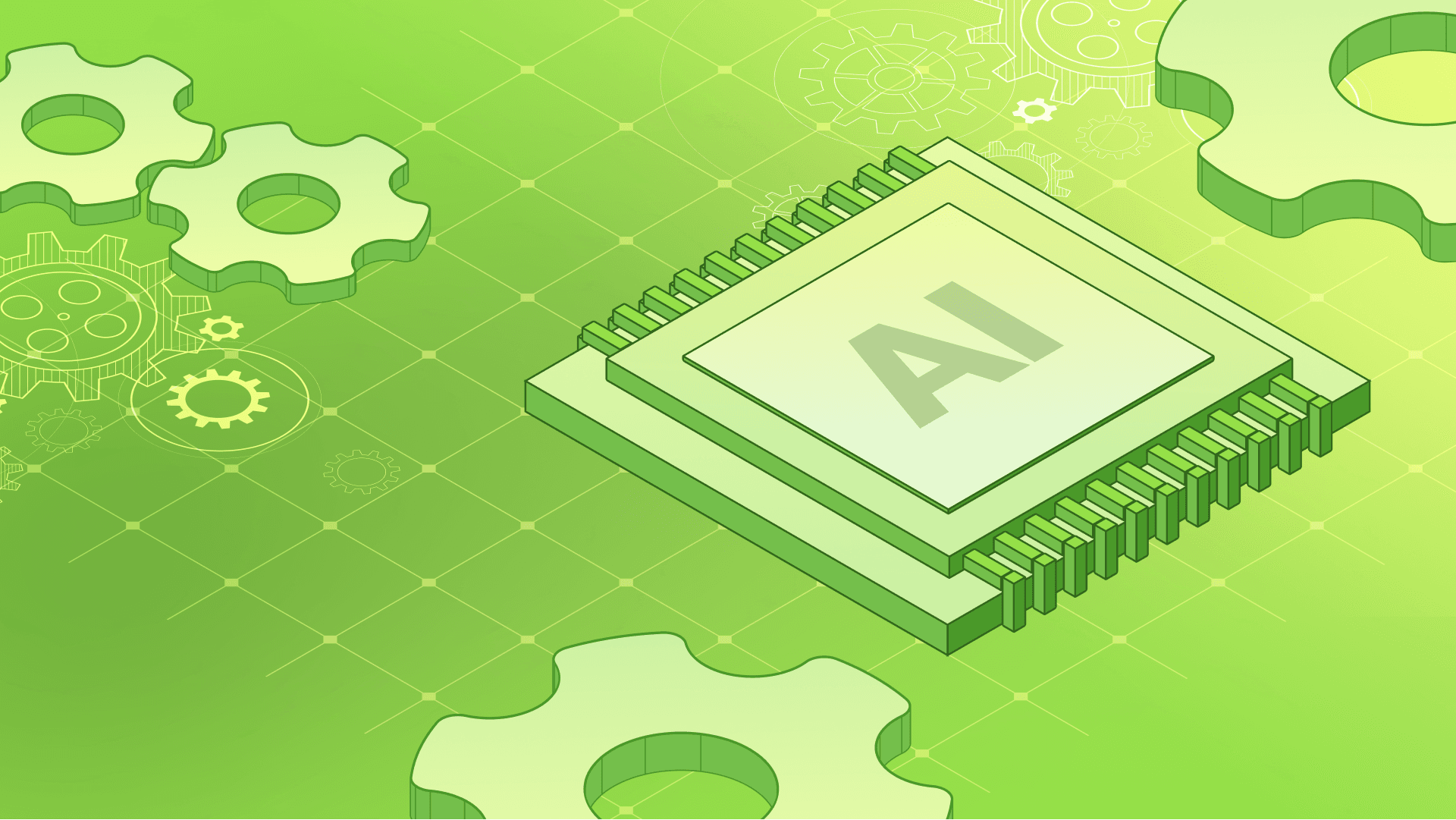
Published on 10/24/2023
Last updated on 02/03/2025
Boosting Machine Unlearning: Model Sparsity Helps You Forget What You Don’t Wanna Remember
Share
Cisco Research has actively engaged in model compression, contributing to this vital field. We are dedicated to supporting efficient AI research at universities and have developed an open-sourced network compression toolbox, ModelSmith.
In a recent collaboration with Sijia Liu from Michigan State University, Cisco researchers, including Yuguang Yao (intern) and Gaowen Liu, have made some new contributions to this field. One of our research papers has been accepted in the prestigious machine learning conference, the 37th Conference on Neural Information Processing Systems (NeurIPS 2023). This work will be shared and presented to the broader research community during NeurIPS 2023 at the New Orleans Ernest N. Morial Convention Center from December 11 - 14, 2023.
This research delves into an exciting and challenging question: is there a theoretically grounded and broadly applicable method bridging the gap between approximate machine unlearning and exact machine unlearning? In this blog post, we embark on a journey to explore the motivation behind Cisco Research's work and delve into the innovative method we have proposed, revolutionizing how we achieve better machine unlearning effectiveness and efficiency via model sparsity by weight pruning.
Introduction
In response to recent data regulation requirements, machine unlearning (MU) has emerged as a critical process to remove the influence of specific examples from a given model. Although exact unlearning can be achieved through complete model retraining using the remaining dataset, the associated computational costs have driven the development of efficient, approximate unlearning techniques. Moving beyond data-centric MU approaches, our study introduces a novel model-based perspective: model sparsification via weight pruning, which can reduce the gap between exact unlearning and approximate unlearning.
We show in both theory and practice that model sparsity can boost the multi-criteria unlearning performance of an approximate unlearner, closing the approximation gap while continuing to be efficient. This leads to a new MU paradigm, termed "prune first, then unlearn," which infuses a sparse model before the unlearning process. Building on this insight, we also develop a sparsity-aware unlearning method that utilizes sparsity regularization to enhance the training process of approximate unlearning.
Question and Solution
We kept asking ourselves, "Is there a theoretically grounded and broadly applicable method to improve approximate unlearning across different unlearning criteria?"
To address this, we advanced MU through a fresh and novel viewpoint: model sparsification. Our key finding was that model sparsity (achieved by weight pruning) can significantly reduce the gap between approximate unlearning and exact unlearning.
Model sparsification (or weight pruning) has been extensively studied, focusing on the interrelation between model compression and generalization. For example, the notable lottery ticket hypothesis (LTH) demonstrated the existence of a sparse subnetwork (the so-called 'winning ticket') that matches or even exceeds the test accuracy of the original dense model. In addition to generalization, the impact of pruning has also been investigated on model robustness, fairness, interpretability, loss landscape, and privacy. In particular, the privacy gains from pruning imply connections between data influence and model sparsification.
Sparsity vs. Unlearning
Prune first, then unlearn. We first analyzed the impact of model sparsity on MU through the lens of unrolling stochastic gradient descent (SGD). When scrubbing a single data point, the specified SGD method allowed us to derive the unlearning error (given by the weight difference between the approximately unlearned model and the gold-standard retrained model). We infused the model sparsity into SGD unrolling. We found that the unlearning error reduces as the model sparsity increases. Furthermore, the weight pruning before unlearning can help improve different kinds of MU methods across different MU metrics.
Sparsity-aware unlearning. We also studied whether pruning and unlearning could be carried out simultaneously without prior knowledge of model sparsity. Considering the unlearning objective function of model parameters and the remaining training dataset after forgetting, we integrated an l1 norm-based sparse penalty into the unlearning process. This led to the sparsity-aware unlearning. We applied a linearly decreasing l1 norm regularization scheduler, and we found that prompting the sparsity during the early stages of unlearning and then shifting the focus to fine-tuning can not only preserve the unlearning efficacy but also ensure the consistent testing accuracy and remaining dataset accuracy compared to the model retraining.
Contributions of the Paper
We share the following contributions in this paper:
∙ First, we provide a holistic understanding of MU across the full training/evaluation stack.
∙ Second, we draw a tight connection between MU and model pruning and show in theory and practice that model sparsity helps close the gap between approximate unlearning and exact unlearning.
∙ Third, we develop a new MU paradigm termed "prune first, then unlearn" and investigate the influence of pruning methods in the performance of unlearning. Additionally, we develop a novel "sparsity-aware unlearning" framework that leverages a soft sparsity regularization scheme to enhance the approximate unlearning process.
∙ Finally, we perform extensive experiments across diverse datasets, models, and unlearning scenarios. Our findings consistently highlight the crucial role of model sparsity in enhancing MU.
Conclusion
In conclusion, the paper accepted at Neurips 2023 introduces an innovative approach to boost the effectiveness and efficiency of approximate machine unlearning, leveraging the power of model sparsity via weight pruning. By combining model pruning and approximate machine unlearning methods, the researchers pave the way for more efficient and effective model unlearning in scenarios where users want their data to be eliminated from the machine learning model. We can look forward to more advancements in the efficiency of achieving exact machine unlearning soon, along with more advanced model pruning-related techniques coming out.
This research constitutes one of the Cisco Research team's numerous outputs. Additionally, our team is actively investigating knowledge distillation, model pruning, model quantization, and various other algorithmic approaches of model compression. We will present our findings systematically and progressively in this area.
To learn more about Cisco Research and other projects we work on and collaborate on, please visit our website, research.cisco.com.
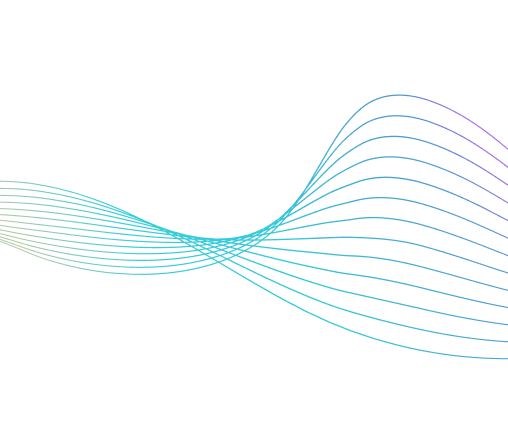
Get emerging insights on innovative technology straight to your inbox.
Welcome to the future of agentic AI: The Internet of Agents
Outshift is leading the way in building an open, interoperable, agent-first, quantum-safe infrastructure for the future of artificial intelligence.
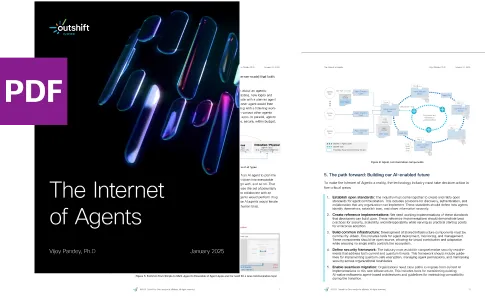
* No email required
Related articles
The Shift is Outshift’s exclusive newsletter.
The latest news and updates on generative AI, quantum computing, and other groundbreaking innovations shaping the future of technology.
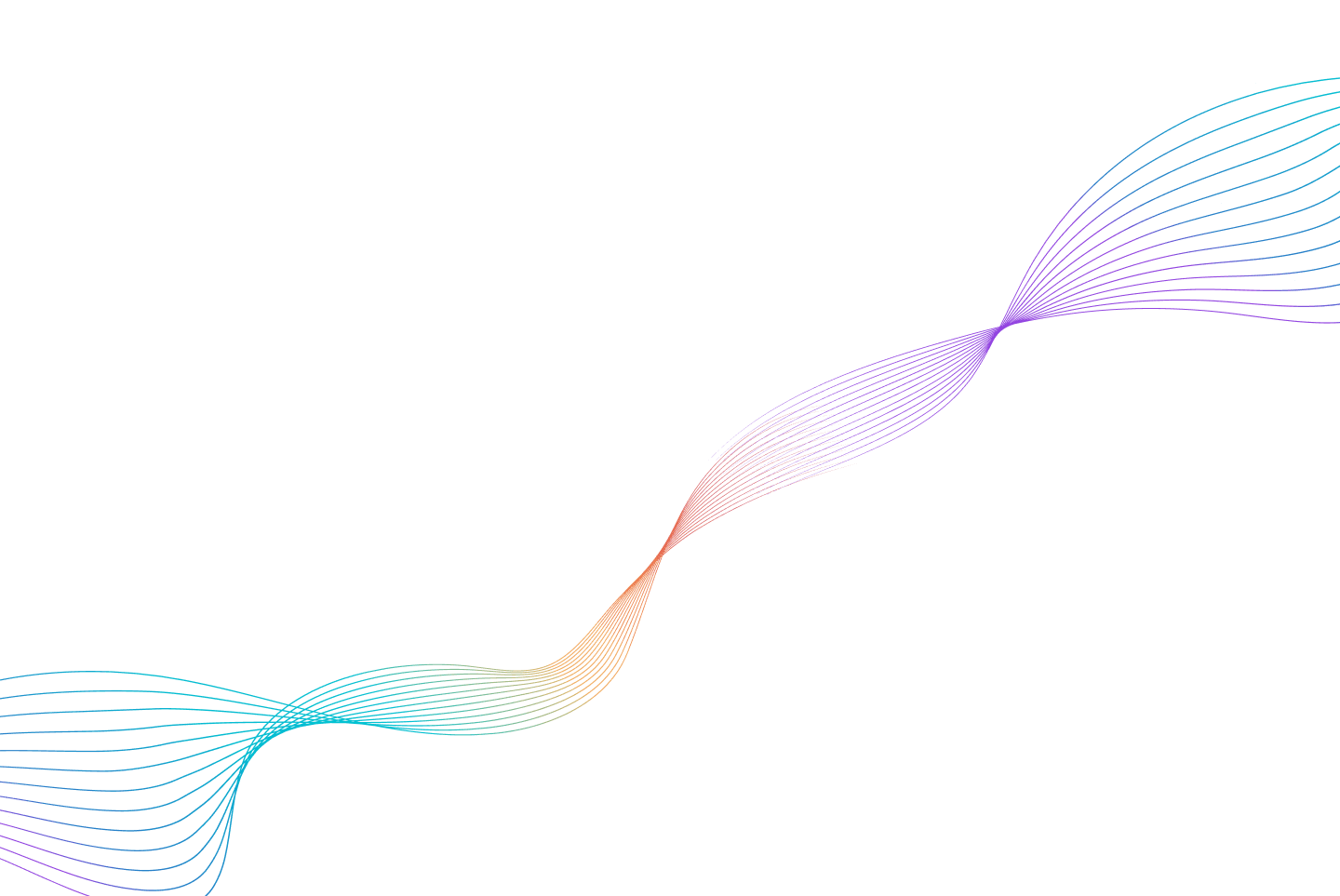