INSIGHTS

5 min read
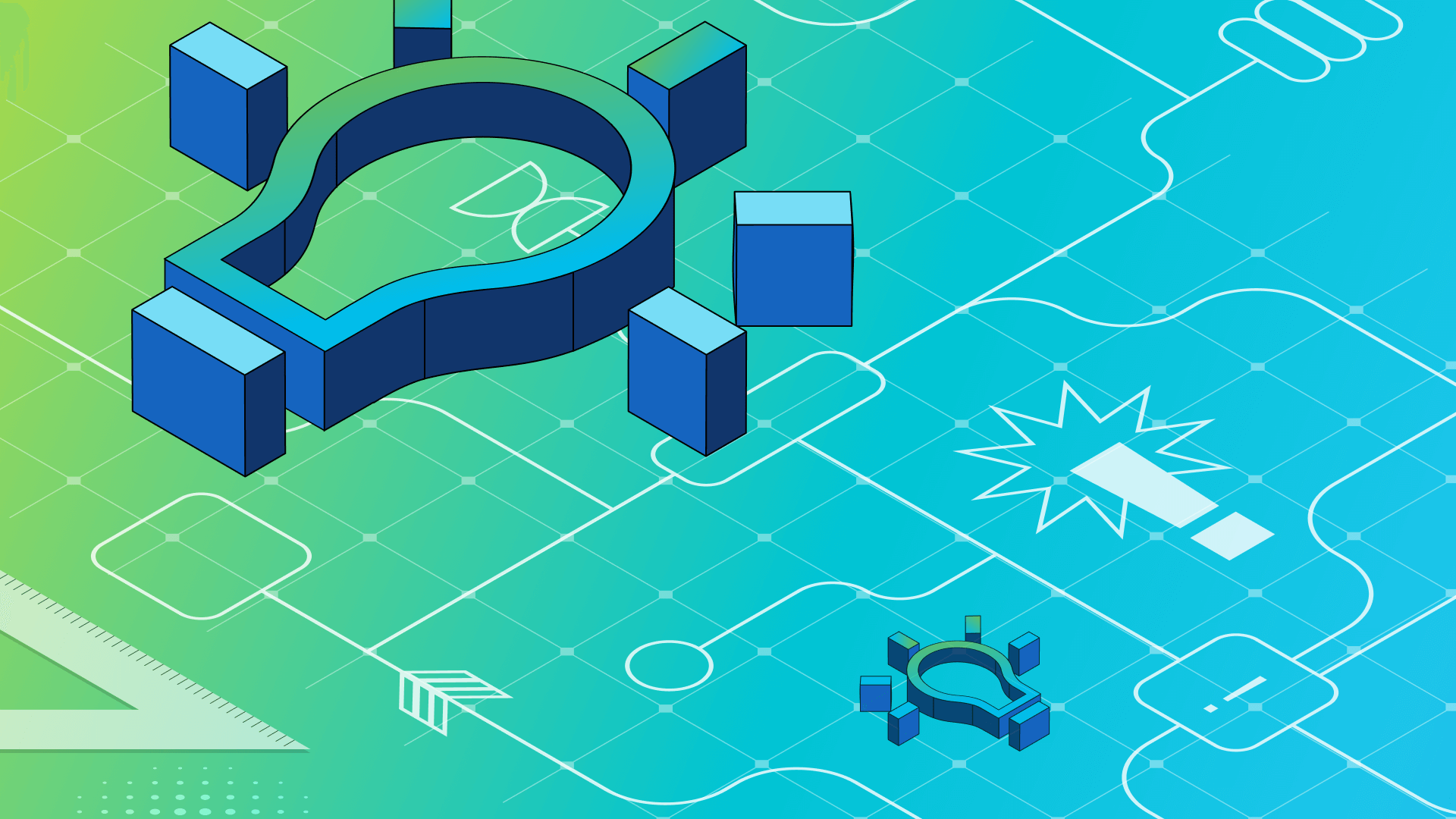
Published on 08/11/2023
Last updated on 02/05/2024
Unleashing the Power of Causality: Introducing Causal Reasoning to Data-Free Model Quantization
Share
Cisco Research has been actively engaged in model compression, making contributions in this vital field. We have been dedicated to supporting efficient AI research at universities and have developed an open-sourced network compression toolbox, ModelSmith.
In a recent collaboration with Bingxin Xu and Yan Yan from the Illinois Institute of Technology, Cisco researchers, including Yuzhang Shang (intern), Gaowen Liu, and Ramana Kompella, have made some new contributions to this field. One of our research papers has been accepted in the prestigious computer vision conference, the International Conference on Computer Vision (ICCV2023). This work will be shared and presented to the broader research community during ICCV 2023 at the Paris Convention Centre from October 2 to October 6, 2023.
This research delves into an exciting intersection where deep learning and causality converge, presenting a novel approach to address a crucial challenge in data-free model quantization. In this blog post, we embark on a journey to explore the motivation behind Cisco Research's work and delve into the innovative method we have proposed, revolutionizing the way we perform model quantization via causal mechanisms without relying on real data access.
Introduction
Over the past few years, deep learning has witnessed remarkable progress in various fields, including computer vision and natural language processing. Researchers have developed network quantization methods to deploy complex deep learning models on resource-constrained edge devices, converting high-precision model parameters into low-precision counterparts. However, a common obstacle in this process is the performance degradation of quantized models compared to their full-precision counterparts. To mitigate this issue, fine-tuning methods are often used to optimize quantized models on complete training datasets. Unfortunately, real-world situations sometimes limit access to original training data due to privacy and security concerns. For instance, patient electronic health records may contain sensitive information and be inaccessible.
Researchers have proposed data-free quantization methods to tackle the challenge of inaccessible training data, enabling model quantization without requiring actual data access. These methods attempt to reconstruct the original data from pre-trained models, leveraging prior statistical distribution information. Despite their effectiveness, these approaches overlook a powerful tool inherent in human cognition: causal reasoning. Humans are adept at learning without extensive data collection, thanks to their ability to perceive causal relationships rather than solely relying on data-driven statistical associations.
In this blog post, we will explore a groundbreaking paper recently accepted at ICCV2023, which introduces causal reasoning to guide data-free quantization. The paper proposes a novel method called Causal-DFQ (Causality-Guided Data Free Network Quantization) to eliminate the reliance on data during the quantized model training. Let us delve into the key insights of this research.
The Power of Causal Reasoning in Data-Free Quantization
Causal reasoning provides a unique perspective on data-free quantization, representing a significant step forward in facilitating data-free network compression. By constructing a causal graph to model the data-free quantization process, including data generation and discrepancy reduction mechanisms, the researchers account for irrelevant factors present in pre-trained models. This approach effectively extracts causal relationships from pre-trained models and disregards irrelevant elements through interventions, emulating how humans use causal language to understand complex scenarios.
Two major challenges are addressed in the paper:
• Constructing an Informative Causal Graph: A fundamental premise for causal reasoning is the construction of an informative causal graph. In a data-free scenario, where real data is unavailable, determining how to construct such a graph has been a challenge in existing literature.
• Formalizing Data Generation and Network Alignment with Causal Language: Connecting causality with data-free quantization relies on formalizing data generation and network alignment using causal language, which remains unresolved in prior work.
Introducing Causal-DFQ: A Novel Quantization Method
• To overcome the aforementioned challenges, the researchers propose Causal-DFQ, a novel method that effectively leverages causality to facilitate data-free quantization. Here is how it works:
• Content-Style-Decoupled Generator: Causal-DFQ introduces a content-style-decoupled generator to synthesize images conditioned on relevant and irrelevant factors (content and style variables). This step ensures that the generated data aligns with causal relationships present in the pre-trained model.
• Discrepancy Reduction Loss: Causal-DFQ incorporates a discrepancy reduction loss to align the intervened distributions of the outputs from pre-trained and quantized models. This process bridges the gap between causal reasoning and data-free quantization, leading to improved model performance.
Contributions of the Paper
The paper's contributions can be summarized as follows:
• Causal Perspective on Data-Free Quantization: The paper pioneers the use of causal reasoning to facilitate data-free network compression, presenting the first attempt in this domain.
• Causal Graph for Data-Free Quantization: By constructing a causal graph, the paper addresses the challenge of informative causal graph construction in a data-free scenario.
• Introducing Causal-DFQ: The novel method, Causal-DFQ, generates fake images conditioned on style and content variables and aligns style-intervened distributions of pre-trained and quantized models, effectively enabling data-free model quantization.
• Empirical Results: Extensive experiments demonstrate the effectiveness of Causal-DFQ in significantly improving the performance of data-free low-bit models. Notably, it surpasses models fine-tuned with real data on the ImageNet dataset.
Conclusion
In conclusion, the paper accepted at ICCV2023 introduces an innovative approach to data-free model quantization, leveraging the power of causal reasoning. By constructing a causal graph and proposing Causal-DFQ, the researchers pave the way for more efficient and secure model quantization in scenarios where training data is inaccessible. This work opens up exciting possibilities for deploying deep learning models on edge devices without compromising on performance. As researchers continue to explore the potential of causality in deep learning, we can look forward to more advancements in data-free quantization and beyond.
This research constitutes one of the numerous outputs produced by our research team. Additionally, our team is actively engaged in investigations on knowledge distillation, model pruning, and various other algorithmic approaches about model compression. We will present our findings systematically and progressively in this area.
To learn more about Cisco Research and other projects we work and collaborate on, please head to our website research.cisco.com.
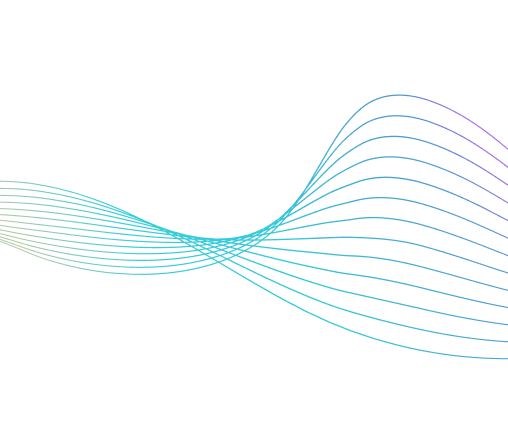
Get emerging insights on emerging technology straight to your inbox.
Unlocking Multi-Cloud Security: Panoptica's Graph-Based Approach
Discover why security teams rely on Panoptica's graph-based technology to navigate and prioritize risks across multi-cloud landscapes, enhancing accuracy and resilience in safeguarding diverse ecosystems.
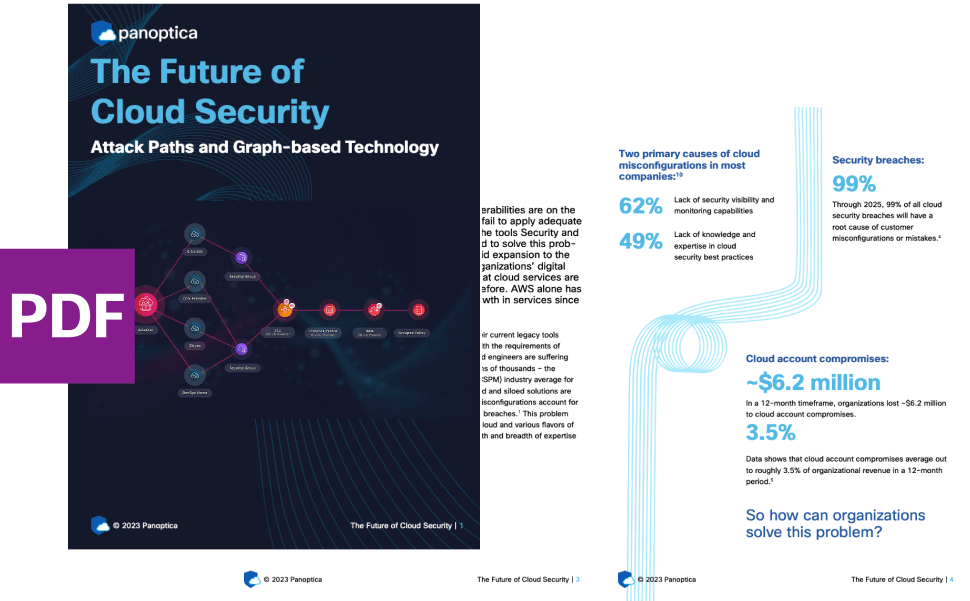
The Shift keeps you at the forefront of cloud native modern applications, application security, generative AI, quantum computing, and other groundbreaking innovations that are shaping the future of technology.
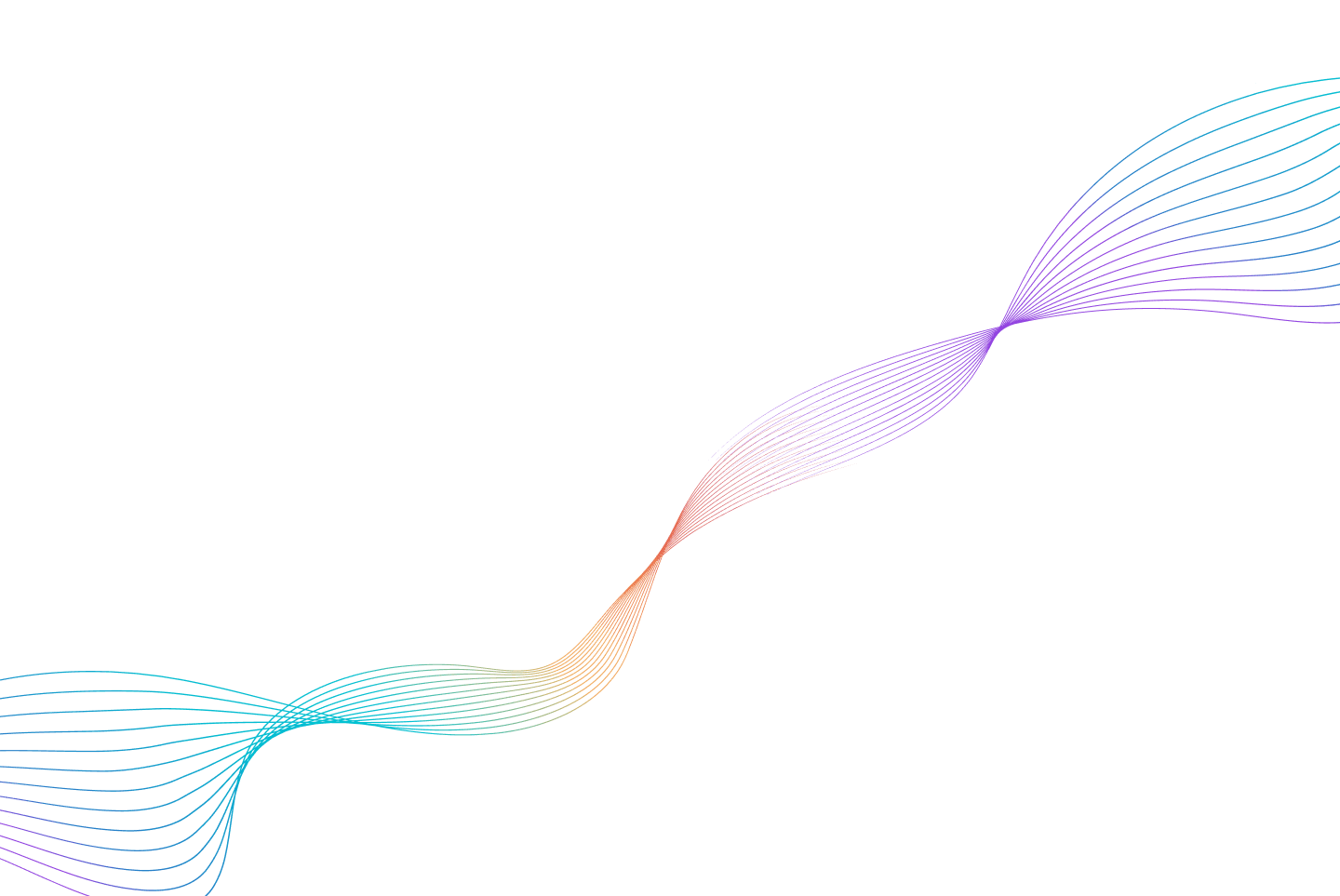