STRATEGY & INSIGHTS

7 min read
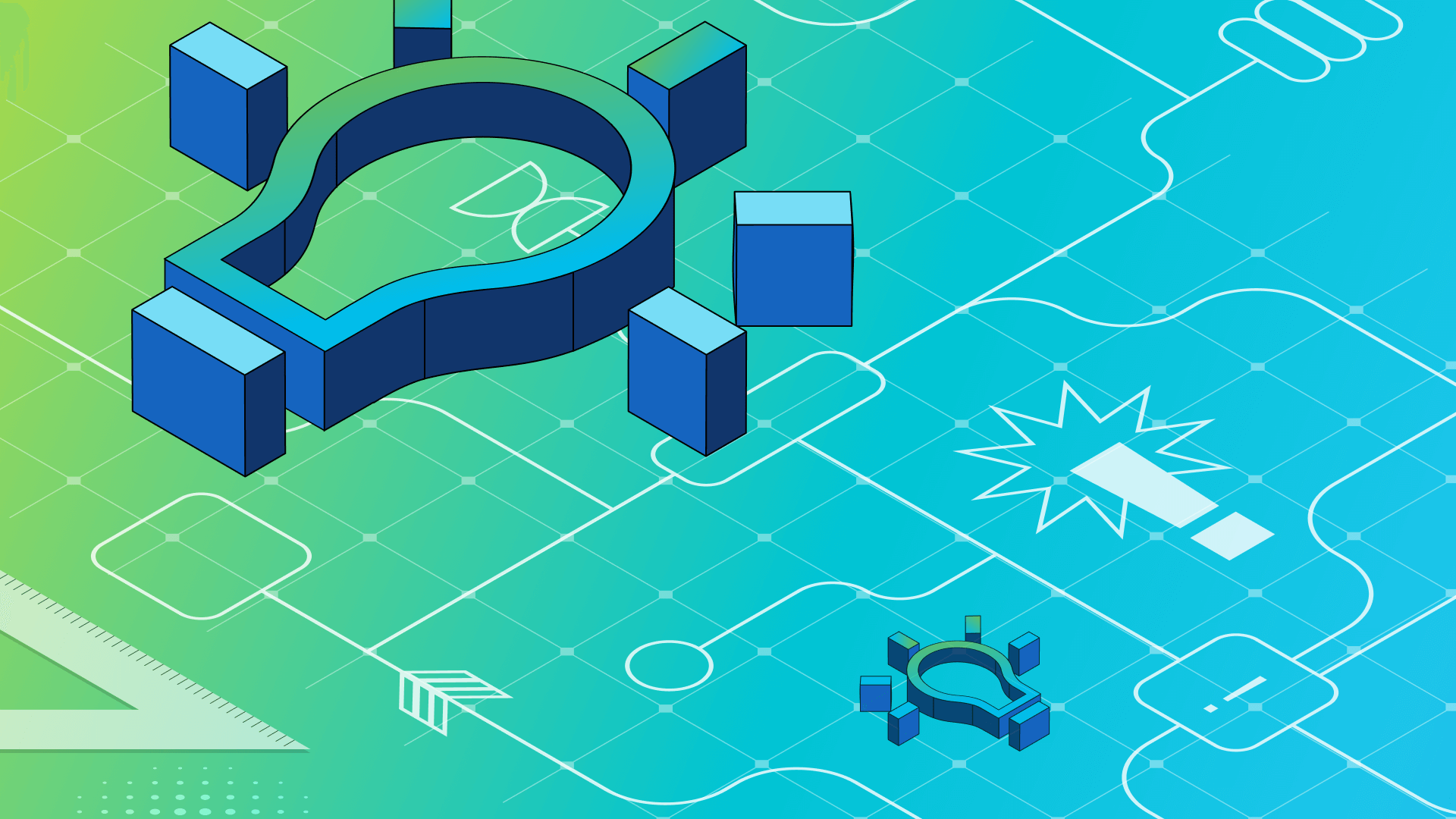
Published on 02/08/2022
Last updated on 02/12/2025
Why artificial neural networks and leadership are not so different
Share
Whether you're a leader looking to enhance your understanding of artificial neural networks through your management experience, a data scientist aiming to leverage your knowledge to step up to senior leadership roles, or simply curious about a fresh perspective on the topic, this post offers valuable insights on artificial neural networks(ANNs), all while saving you the time and effort of extensive reading.
What is an artificial neural network?
First, the basics: artificial neural networks (ANN) is a series of connected nodes called artificial neurons, loosely designed to model the connections in a human brain. Companies use artificial neural networks for predictive modeling and adaptive control—and any application where a dataset can help enhance AI training.
Artificial neural networks are a powerful AI approach that allows computers to learn from input data without being explicitly programmed. The neural network draws inspiration from the biology of the brain and can learn on its own, making machines more human-like, transmitting information between layers of so-called artificial neurons., better known as Artificial Neural Networks (ANN).
In short, ANNs are to help machines learn: in this context, a network can learn from context and experience. Over a long enough period, the lessons an artificial neural network absorbs may even start to feel like leadership.
Using the TechGrowthMindSet to learn new technologies
As professionals, we constantly learn and adapt to new technologies and methods to improve our skills and stay ahead of the curve. However, the task of learning something unfamiliar can be daunting and overwhelming. This is where the #TechGrowthMindSet, an interdisciplinary approach, comes into scope.
The #TechGrowthMindSet approach involves finding connections between new technologies and other aspects of our personal and professional lives. It may even mean embracing failure as a learning model. This method allows us to learn new technologies efficiently and gain insights into how they can be applied in various areas of our lives.
For instance, in industries where AI/ML and neural networks are leveraged to deliver robust and transformative solutions, practitioners can use the #TechGrowthMindSet approach to understand the technology better. By asking questions such as :
"What do I already know that can be applied to ANNs?" and "How can this knowledge help me better understand the functionality of ANNs?"
By inquiring about the applicability of existing knowledge to ANNs and seeking ways to better comprehend their functionality, you can efficiently obtain valuable insights to propel innovative solutions forward. In the following section, you can leverage your leadership experience to navigate the intricate aspects of artificial neural networks.
Connecting the dots: Artificial neural networks and leadership
Architecting and deploying an artificial neural network is akin to practicing a successful leadership strategy. Many of the foundational principles are quite similar. For instance, artificial neural networks and successful leadership strategies rely on feedback-based adaptive learning. Leaders who seek feedback from their customers and teams can improve business outcomes, just as neural networks depend on feedback loops to deliver valuable insights.
Moreover, a good neural network has to be efficient, accurate, verifiable, robust, ethical, and tamper-proof. In contrast, a good leadership approach has to be nimble, scalable, and effective yet maintain transparency and integrity. Additionally, just as good leaders can thrive in the messiness of navigating ambiguity and leading change within organizations to deliver results, artificial neural networks outperform machine learning techniques when dealing with messy volumes of unstructured data such as images, audio, and text.
If you are familiar with the basics of neural networks, skip the introductory section and dive straight into the next section.
Introduction to artificial neural networks
As breakthroughs in artificial neural networks have made way for deep learning in recent years, companies across all industries are implementing this technology as part of their AI strategy. Deep learning has enabled a wide range of new advanced smart services in consumer and industrial applications, from sentiment analysis in customer service to image recognition in retail, surveillance, autonomous vehicle driver safety, healthcare, etc.
ANNs take a layered approach to processing information and making decisions. In its basic form, an ANN can have only three layers of neurons: the input layer (data ingestion point), the hidden layer (processing point), and the output layer (decision point). ANNs can often get more complex with multiple hidden layers. We refer to it as deep learning when there are more than three layers. Whether it’s three layers or more, information flows from one layer to another, creating a relay of information flow. Deep artificial neural networks are a set of algorithms that have set new records in accuracy.
For a neural network to learn, there must be an element of feedback involved—similar to humans learning by being told what they are doing right or wrong. So, once you have trained the artificial neural network with enough learning examples, it reaches a point where you can present it with an entirely new set of inputs it has never seen before and examine how it responds. Amazing right?
Artificial neural networks: A leadership approach in 12 steps
To better understand the relationship between artificial neural networks (ANNs) and successful leadership strategies, it can be helpful to break down the components of ANNs at various phases of the development process and compare them to leadership actions. This can be done in a simple step-by-step approach, as shown in Figure-3 below.
Each action of an ANN corresponds to a leadership action shown on the right. It's worth noting that this process on the left generally applies to designing AI/ML models, not limited to neural networks. However, feedback loops are predominantly characteristic of ANNs and are an important component of effective ANNs and successful leadership strategies.
If this fresh outlook of examining ANNs through the lens of leadership has proven informative and valuable to you, we will delve into each step in more detail in future posts. Furthermore, it's important to note that the #TechGrowthMindSet goes both ways, and leaders can also learn from the efficiency of ANNs. It's not just about humans teaching machines but also about reflecting on what we can learn from technology and machines.
It would be interesting to see if leaders consider evaluating their leadership approach based on the insights gained from learning about ANNs to enhance their current practices.
Applying artificial neural network principles to continued growth in leadership
In conclusion, applying artificial neural network principles to leadership can lead to more efficient and effective outcomes. By drawing parallels between these two disciplines, we can gain insights that apply to personal and professional growth. As AI and machine learning continue to play an increasingly important role in organizations, embracing a growth mindset and actively seeking opportunities to learn and improve is essential. I encourage you to share your experiences and insights in the comments as we continue to explore the exciting possibilities of this rapidly-evolving field.
What’s next? If you want to understand more about embracing an uncertain future, we recommend you read Harnessing Fear for Professional Growth.
Pallavi Kalapatapu is a Principal engineer and open source advocate in Cisco’s Emerging Technology & Incubation organization, now Outshift by Cisco.
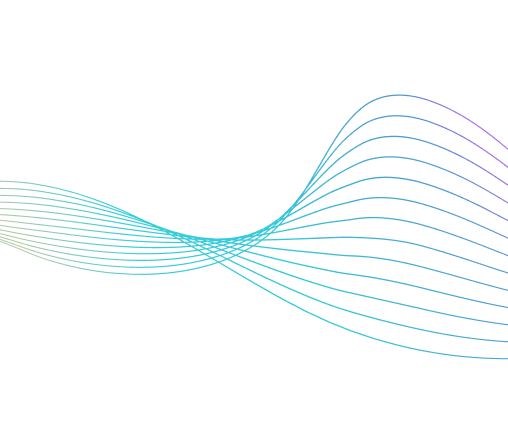
Get emerging insights on innovative technology straight to your inbox.
Welcome to the future of agentic AI: The Internet of Agents
Outshift is leading the way in building an open, interoperable, agent-first, quantum-safe infrastructure for the future of artificial intelligence.
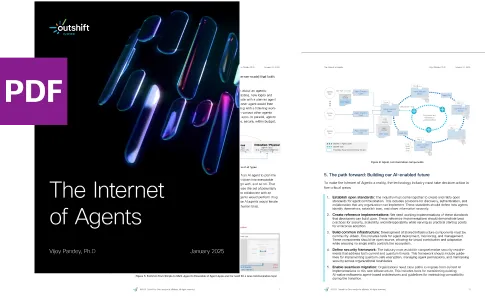
* No email required
The Shift is Outshift’s exclusive newsletter.
Get the latest news and updates on agentic AI, quantum, next-gen infra, and other groundbreaking innovations shaping the future of technology straight to your inbox.
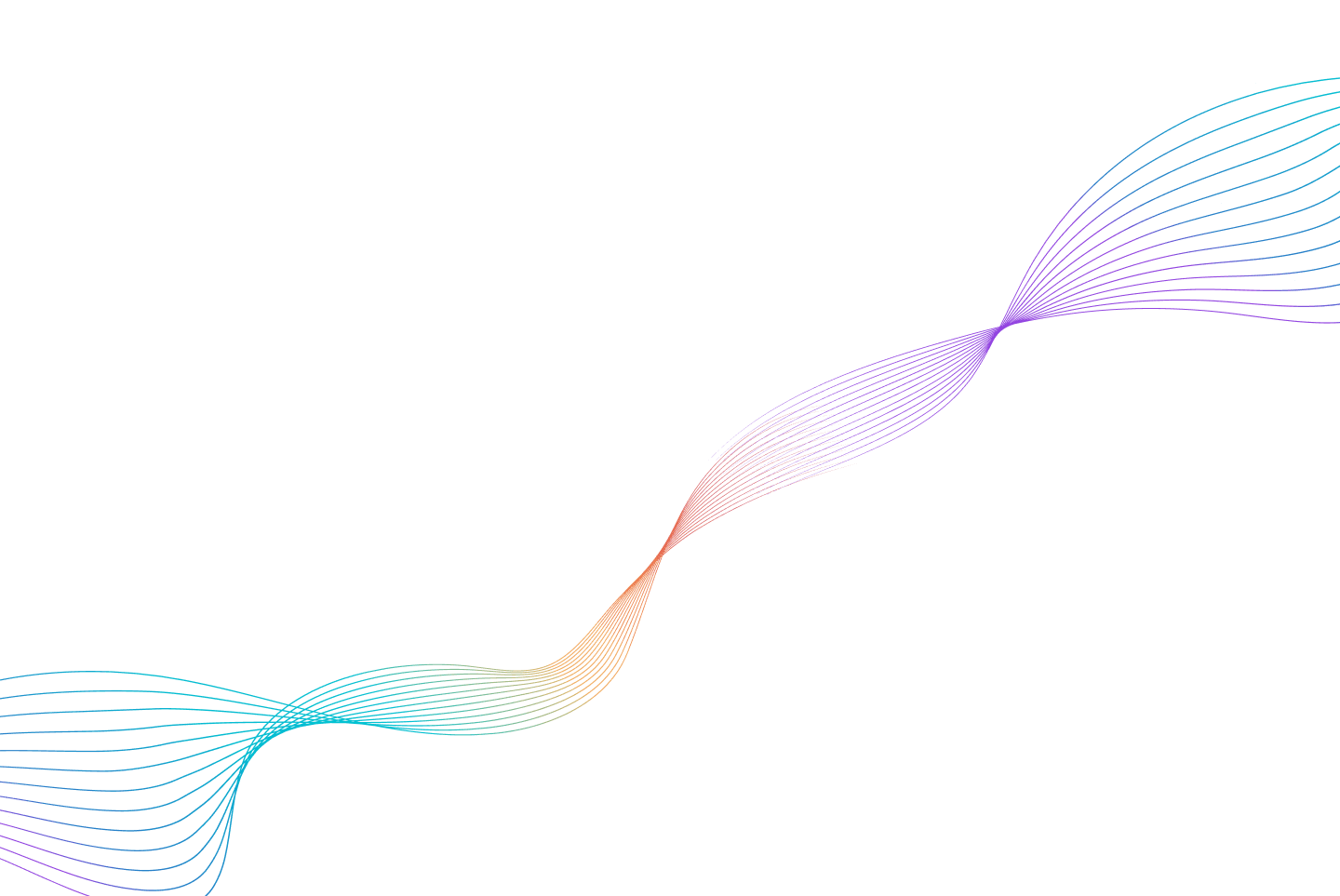