INSIGHTS

10 min read
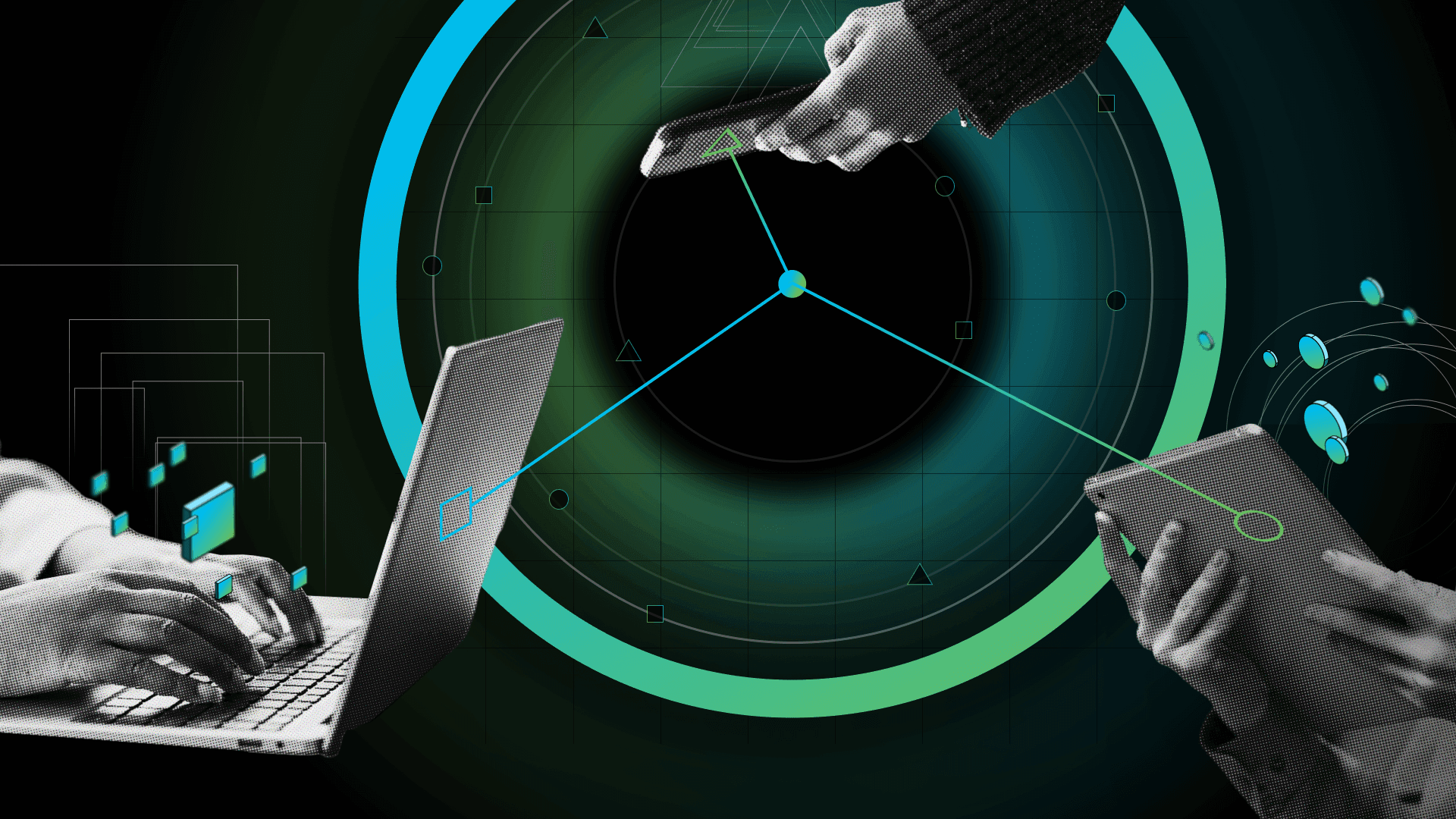
Published on 01/05/2023
Last updated on 06/18/2024
Generative AI to redefine ‘info workers’ and turn ‘full stack observability’ on its head in the process…
Share
An information worker is someone who works primarily with information and knowledge, rather than with physical goods or materials. This includes tasks such as research, analysis, communication, and problem-solving, often using computers and other digital tools. Information workers are found in a wide range of industries and sectors, including business, finance, education, healthcare, and government. Some common examples of information workers include executives, analysts, journalists, teachers, and researchers. In many cases, information work requires a high level of education, skills, and expertise, and is often associated with knowledge-intensive industries. Information workers are sometimes also referred to as "knowledge workers."
Full stack observability is the ability to monitor and understand the performance and behavior of a system or application at every level, from the front-end user interface to the back-end infrastructure and data stores. It involves collecting and analyzing data from various sources in order to gain visibility into the system's health, performance, and usage patterns. Generative AI is a type of artificial intelligence that is capable of generating novel and often highly realistic outputs, such as images, text, and even computer code. It is typically achieved through the use of machine learning algorithms, which are trained on large datasets and can learn to generate new content that is similar in style or content to the input data.
Generative AI has a wide range of potential applications, including creative tools, data analysis, and automation.
Welcome! to the era of generative AI and its potential to revolutionize the way we think about information workers and full stack observability. In recent years, generative AI has garnered significant attention for its ability to generate novel and often highly realistic outputs, such as images, text, and even computer code. But beyond its capabilities as a creative tool, generative AI also has the potential to fundamentally change the way we approach tasks and workflows in a variety of industries. In this post, I'll touch on how generative AI is set to redefine the role of 'info workers' and turn the concept of 'full stack observability' on its head in the process.
More specifically, generative AI has transformative potential in the area of 'Low code and no code' tools which allow users to build and deploy software applications without the need for extensive coding knowledge. These tools are designed to be user-friendly and intuitive, and often include pre-built templates and drag-and-drop interfaces that make it easier to create custom applications and integrations. Low code - no code tools are all about increasing the productivity of knowledge workers, particularly in fields where there is a need and opportunity to quickly build and deploy custom applications or integrations. By eliminating the need for complex coding, low code and no code platforms allow users to focus on the design and functionality of their applications, rather than spending time on the underlying technical implementation.
A real-world example of low code no code use case is AppSheet. AppSheet is a cloud-based platform that allows users to build custom mobile and web applications without writing any code. AppSheet has been used by a company called Field Nation, which provides a platform for connecting businesses with freelance technicians for on-site repairs and installations. Field Nation used AppSheet to build custom mobile apps for its technicians, which allow them to access job assignments, track their progress, and submit invoices and other documentation directly from their phones. By using AppSheet, Field Nation was able to streamline its operations and improve the efficiency of its technicians, leading to significant time and cost savings. And it was not that long ago that a company of Field Nation 'class' could not even contemplate that level of customization without embarking on a multi-year, multi $$ outsourced IT endeavor.
However, one drawback of low code and no code tools is that they may not provide the same level of control or customization - freedom of coding really - as traditional coding approaches. By relying on pre-built templates and libraries, users may be limited in terms of the specific features and functionality that they can incorporate into their applications. Moreover, even with their visual and template-based approaches, the heart of the coding process is still articulated in a pseudo-coding structure and manner - still I myriad of flows, loops, data arrays and what not. In short, traditional low code - no code is only a half-measure towards the true democratization of the gift of code writing.
The use of natural language to code, also known as natural language programming or natural language processing, refers to the ability of a computer system to understand and execute instructions written in a natural language, such as English, rather than a programming language. This type of technology has the potential to revolutionize the way we think about coding and programming, as it allows non-technical users to create custom software and automate tasks without the need for extensive coding knowledge. One of the key benefits of natural language programming is that it can significantly increase the accessibility and usability of coding and programming for non-technical users. With a language that is familiar and intuitive, users can more easily understand and execute instructions, reducing the learning curve and making it easier for them to get started. By using a more intuitive and expressive language, subject-matter-experts-turned-developers can more easily communicate their intentions and ideas, leading to more efficient and effective creation, integration and collaboration. This can help to reduce the time and effort required to build and maintain software applications, leading to significant, perhaps dramatic productivity gains. According to the U.S. bureau of labor statistics, measuring employee productivity means calculating 'output per hour' of work. However, in the knowledge economy, each person is an asset bringing their own particular expertise, experiences and ideas to the table. The expression of that knowledge is accomplished with tools given. Those tools are what drive output, which in turn translate to commercial and financial gain.
The following diagram depicts the typical time and mind share allocated by info. workers in their daily routines:
That upper combined 70% of that precious stack where info. workers basically ‘do what they do’ (desk work) and integrate what they do across their respective orgs. (manage across) is the knowledge worker green-field of optimization and opportunity which could be tapped into by unleashing the era of ‘tools created’ by workers, rather than ‘tools given’. The inability to directly link financial outcomes to the efficiency of knowledge work calls into question many of the standard operational metrics used to gauge the productivity of software engineering teams, accounting groups, call centers, etc. If financial metrics are first order measures of company performance and operational metrics are second order measures, then employee activity, time utilization and job satisfaction metrics are at least the tertiary factors in determining business success. This grand act of info. workers empowerment is already underway. One needs only consider the centricity, usage and role of a ‘legacy productivity’ app. such as Microsoft Excel, and look at what generative AI tools such as ‘excelformulabot’ (excelformulabot.com) make possible to appreciate the potential yield of this step-change transformation.
Phew! If you made it this far in this blog post, I’m going to assume that I have you at least partially sold on the magnitude of the potential encapsulated in the unfolding vision of subject-matter-experts-turned-developers. All them SMEs, in all them enterprises buzzing round their daily chores now talking to their PCs and phones, creating (!) and refining a perpetually expanding and deepening NEW set of applications reaching into and between the IT resources available to them like never before. Never before! But, you may wonder, what does this brave new world of generative AI, and this newly formed army of enterprise coders has to do with…full stack observability?! What does a x10, x100…x1000 fold increase in the number of coders in a given enterprise has to do with FSO? How may it impact the carefully laid and woven monitoring and logging, consolidating and gathering of data about all the components of a system in order to understand and optimize its behavior? The hardware, the operating systems, the network, the user experience and the application’s code…? By way of over generalization, the standard practice of an effective FSO solution is relegated to the ingestion of disparate logs, events and patterns inconsistently exposed by IT resources and systems employed, which I’m going to generally refer to as ‘IT exhaust fumes’. In a more favorable, more open, less heterogeneous IT setting, which is increasingly NOT the norm – hence the increasing need for FSO in the first place – in-house and vendors implementation of good instrumentation and telemetry in ‘your’ code, which may include adding code to emit logs, metrics, and traces that provide insight into the inner workings of your IT operations…is the methodical path to truly effective FSO. Much favorable than the ingestion and backwards engineering of ‘ IT exhust fumes’. Is it not?
Here are several challenges that arise when implementing full stack observability: • Data availability: data may not always be available when collecting data from multiple sources, including 3rd party proprietary sources at different layers of the system. • Data overload: Collecting data from all layers of the system can generate a large volume of data, which can be difficult to manage and analyze. This can make it difficult to identify the most important issues and prioritize them. • Integration and compatibility: Different monitoring and logging tools may use different data formats and protocols, which can make it challenging to integrate them and get a unified view of the data. • Privacy and security: Collecting data about users and their interactions with the system raises privacy and security concerns. It's important to be transparent about what data is being collected and how it is being used, and to implement appropriate security measures to protect sensitive data. Resource overhead: Monitoring and logging can add overhead to the system, which can impact performance. It's important to strike a balance between the level of monitoring and logging needed to get useful insights and the impact on system performance. • Culture and process: Adopting a full stack observability approach requires a shift in culture and process, as it requires collaboration and cross-functional communication across different teams. This can be a challenge for organizations that are used to working in silos.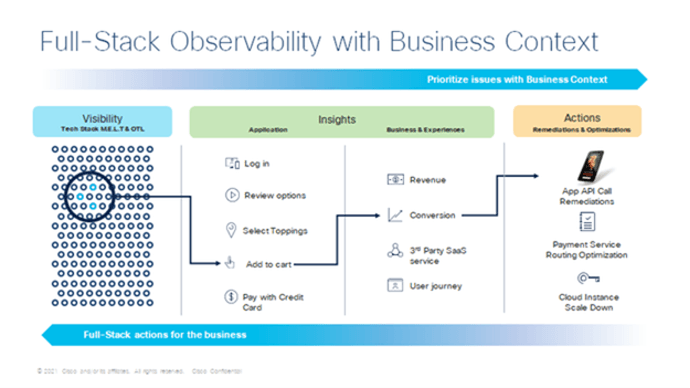
The thesis, the trend-to-watch-and-act-upon, therefor, is that generative AI is leading to a step-change in the quantity and quality of ‘in-house’ code. A LOT more code, and a LOT more integration and utilization of IT resources, expressed at a high-level – as high as spoken language and SME intent levels – will increasingly act as an extensive dynamic ‘mapping’ of the enterprise IT complex. The data stores, the business processes, the interdependencies, the analytics, the causes and effects etc. could all be organically surfaced, accessed and employed for FSO which is as effective, powerful and impactful as the newly grazed pastures of knowledge workers which will soon code all day long.
The bottom line 1,2,3s of this all might then be:- Consider the investment in, access to and ownership of code generative AI tools and platforms
- Consider the standards and hooks into 3rd party code generative AI so it may be also be leveraged for effective FSO
- Architect today for FSO that is based on a code generative AI fabric future, rather than 'IT exhaust fumes' alone
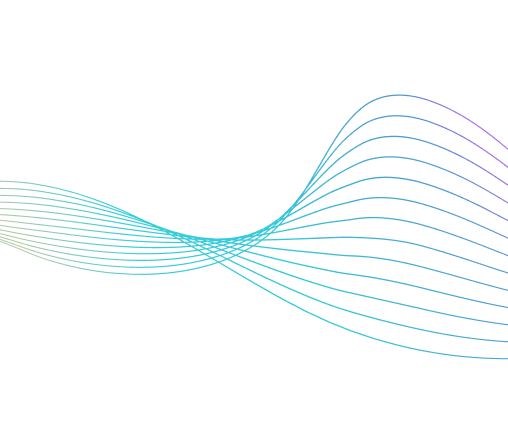
Get emerging insights on innovative technology straight to your inbox.
Unlocking multi-cloud security: Panoptica's graph-based approach
Discover why security teams rely on Panoptica's graph-based technology to navigate and prioritize risks across multi-cloud landscapes, enhancing accuracy and resilience in safeguarding diverse ecosystems.
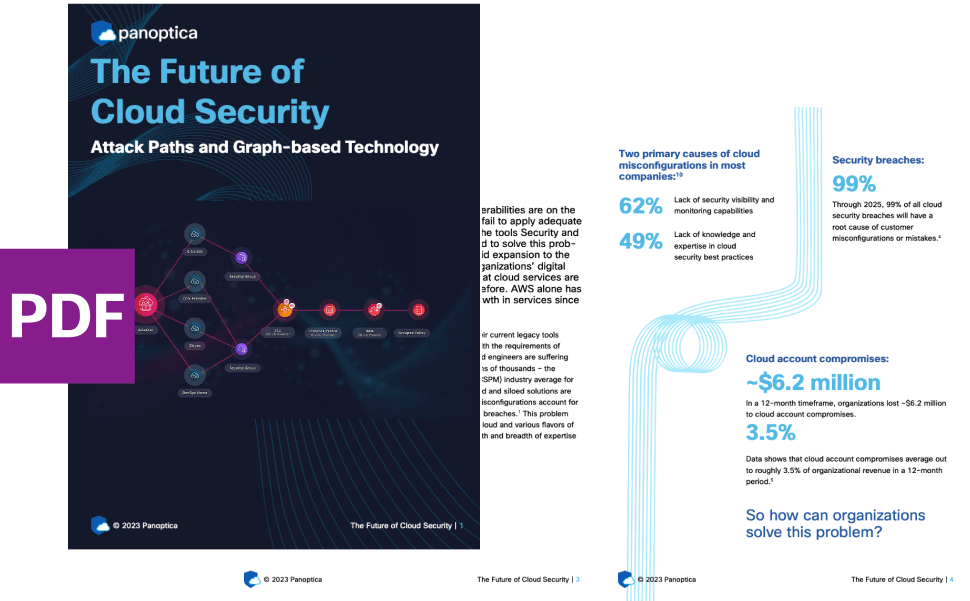
Related articles
The Shift keeps you at the forefront of cloud native modern applications, application security, generative AI, quantum computing, and other groundbreaking innovations that are shaping the future of technology.
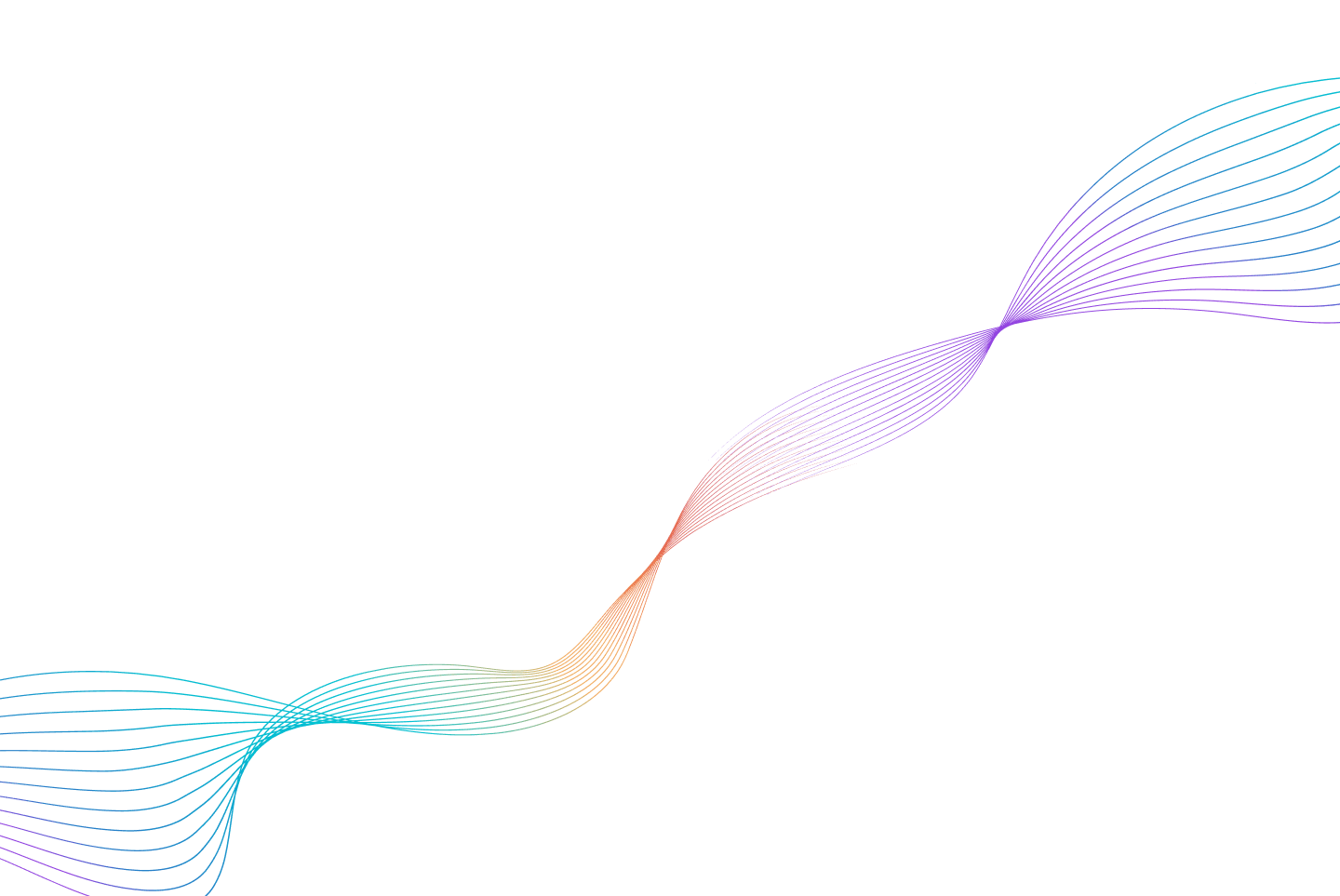