IN-DEPTH TECH

4 min read
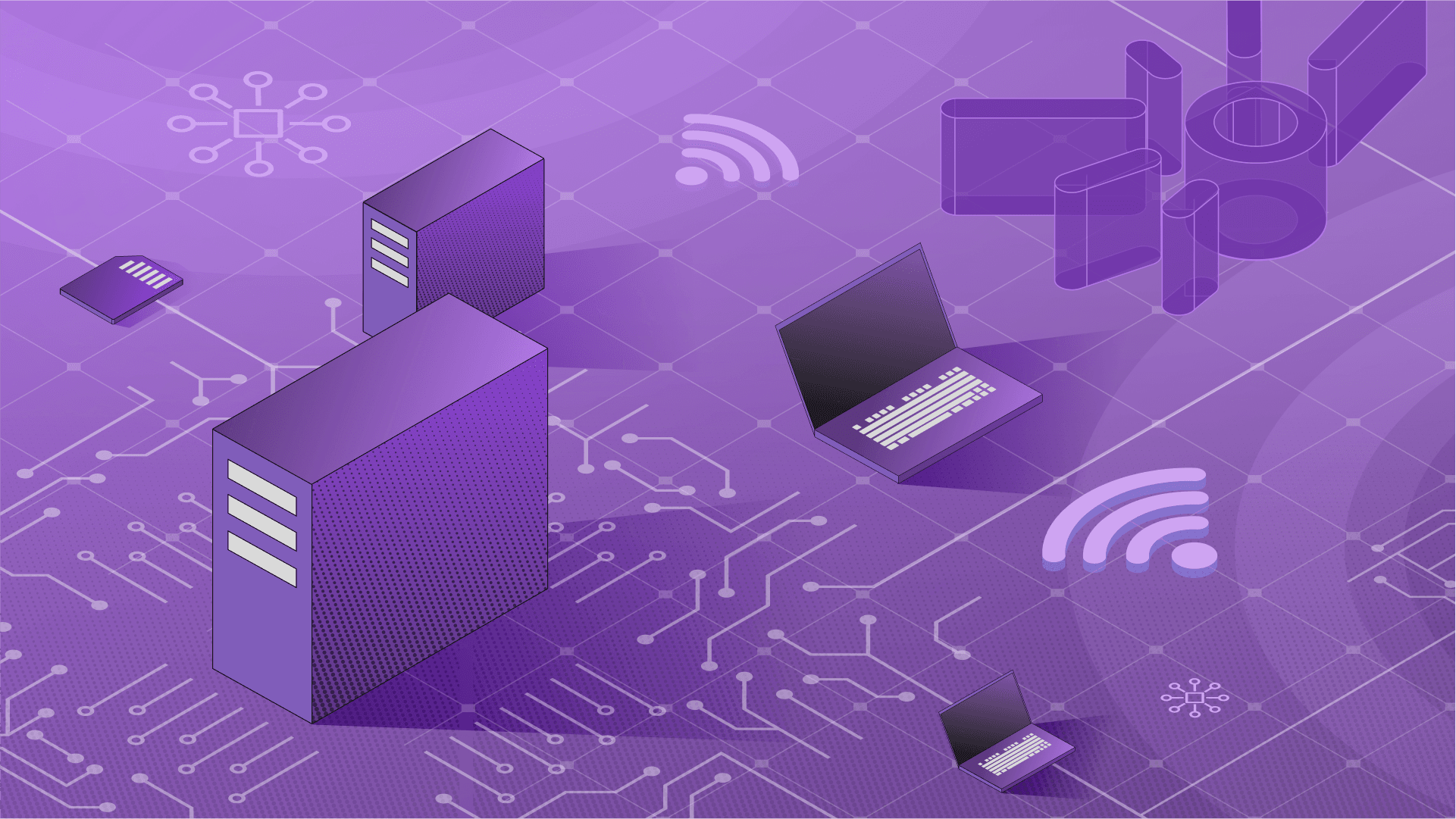
Published on 07/06/2021
Last updated on 02/12/2025
AI 2.0 - Episode #1, Introduction
Share
There is a growing realization in academia and industry that that the state-of-the-art in artificial intelligence, namely deep learning, is flawed but remains quite useful in many domains ranging from conversational agents, demand forecasting to recommendations and more. Hinton, who is thought of by many as "the Father of Deep Learning", has stated that in order to achieve a higher level of capability, we “need to start over”. It seems every day a new business leader or luminary, even the normally very positive Woz has viciously attacked deep learning based AI saying there is nothing intelligent about it. Deep learning achieves high marks for hype by Gartner who also claim that more than 67% of deep learning projects fail.
It is doubtful that this is new information to anyone reading this blog. AI is currently used to provide recommendations, power your speech-enabled digital assistants, and much more. While these systems do provide value, most people would agree that, based on first-hand experience, deep learning leaves room for improvement.
Another consideration is that deep learning systems can be extremely difficult, expensive, and resource intensive to train. For example, OpenAI’s GPT-3 language model costs more than $4M to train at an estimated > 1GWh of energy. Of course, most deep learning models don’t require anywhere near this level of resource, however even this enormously powerful language model gets dismal marks in terms of natural language understanding tasks as emphasized by Yan Lecun:
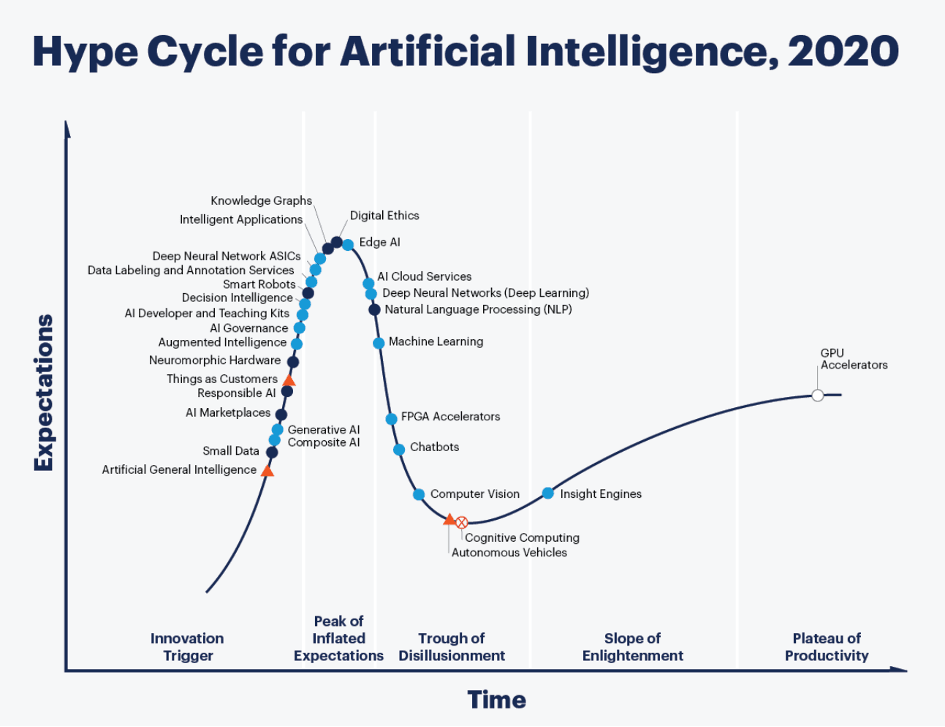
“… trying to build intelligent machines by scaling up language models is like building high-altitude aeroplanes to go to the moon.”For a deeper understanding of the source of these limitations, please see Google’s late 2020 paper:
Underspecification Presents Challenges for Credibility in Modern Machine LearningIn essence, the paper proves what many deep learning practitioners and academics already knew but couldn’t prove. Namely that simply increasing the size of deep learning systems in terms of layers and nodes does not necessarily lead to more intelligent systems. Beyond the well-known overfitting problem, what results are systems which appear to work extremely well during training and cross validation testing but completely fail in the field. One solution to this is to begin to analyze and understand the inductive bias (i.e. On what basis does a system generalize?) of these systems. Another solution to these problems being explored by the AI community is known as Deep Learning 2.0 or as Bengio termed it: “Deep Learning for System 2 Processing” referring to Daniel Kahneman’s terminology where system 1 is defined as: fast, unconscious, automatic, effortless and system 2 is defined as: slow, deliberate, conscious etc… thinking. Deep learning 2.0 can be thought of as the incorporation of symbolic AI, for example: knowledge graphs, concepts, causal reasoning, significantly improved generalization and more into the deep learning paradigm. This approach is already resulting in greatly improved results on many tasks as exemplified by Hinton’s GLOM and Bengio’s causal reasoning work.
The future of neural networks is Hinton's new GLOM model Yoshua Bengio Team Proposes Causal Learning to Solve the ML Model Generalization ProblemOur AI 2.0 approach (https://www.researchgate.net/project/A-Metamodel-and-Framework-For-AGI) builds on these developments with a neurosymbolic architecture that includes a formal model of knowledge as well as intrinsic large-scale time series processing capability. Our knowledge model supports levels of abstraction, symmetric and anti-symmetric relations, while the large-scale time series functionality includes zero-shot learning of structure and one-shot learning of associated natural language. We have found that these seemingly minor additions lead to remarkable results. For example, cumulative learning, zero-shot and one-shot learning, the holy grail of AI, are emergent properties of systems based on our AI 2.0 technology. This series of blog posts will dive into Deep Learning 2.0, AI 2.0, and our applications of AI 2.0 to projects such as SensorDog and Kronos.
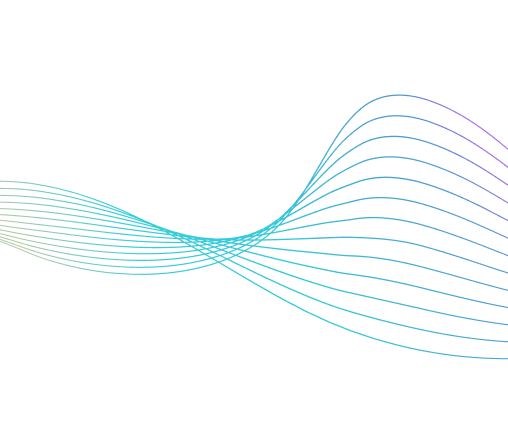
Subscribe to
the Shift!
Get emerging insights on innovative technology straight to your inbox.
Welcome to the future of agentic AI: The Internet of Agents
Outshift is leading the way in building an open, interoperable, agent-first, quantum-safe infrastructure for the future of artificial intelligence.
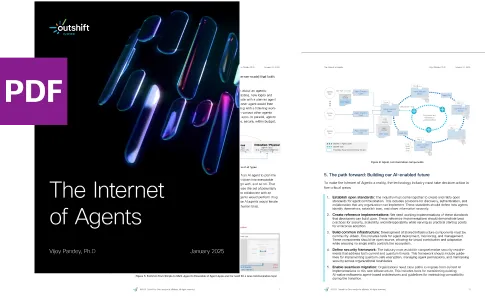
* No email required
Subscribe
to
The Shift
!Get on innovative technology straight to your inbox.
emerging insights
The Shift is Outshift’s exclusive newsletter.
The latest news and updates on generative AI, quantum computing, and other groundbreaking innovations shaping the future of technology.
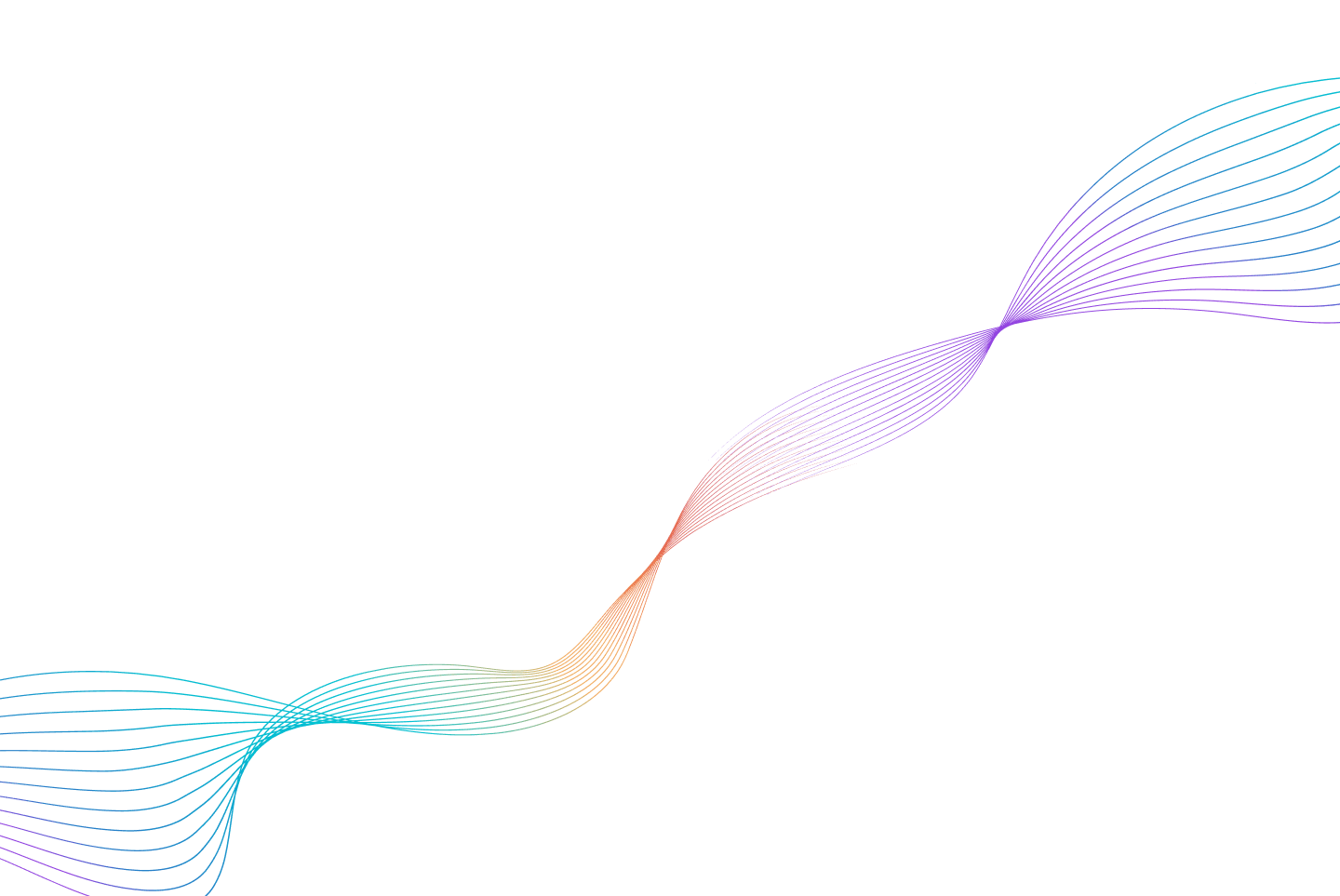